|Workshop| Machine learning for buildings energy management
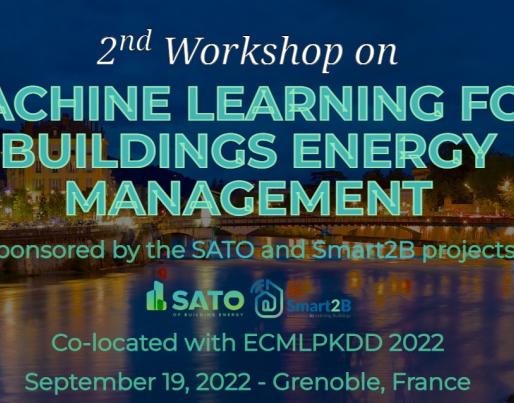
|Workshop| Machine learning for buildings energy management
Relevance to the Machine Learning Community
Energy efficiency is of the utmost importance for modern societies and for the sustainability of our planet. European buildings remain predominantly inefficient, accounting for 40% of final energy consumption and 36% of the total EU CO2 emissions. EU targets for 2030 include reaching a 32% share of renewable energy and increasing energy efficiency by at least 32.5%. Due to the scale and complexity of current building energy systems, traditional modeling, simulation and optimization techniques are feasible and unable to achieve satisfactory results. To achieve the aforementioned goals, modern buildings require the capabilities of self-assessing and self-optimizing energy resources, of meeting user preferences and requirements, and to contribute to an overall better and sustainable energy system. Based on the recent history, we plan to organize this workshop as a European forum for building’s energy and ML researchers and practitioners that wish to discuss the recent developments of ML for developing BEMs, by paying special attention to solutions rooted in techniques such as pattern mining, neural networks and deep learning, probabilistic inference, stream learning and mining, and big data analytics and visualization.
Motivation
Increased energy efficiency and decarbonization of the energy system are two primary objectives of the European Energy Union. These objectives are clearly demonstrated in ambitious EU targets for 2030, namely, reaching a 32% share of renewable energy and increasing energy efficiency by at least 32.5%. Meanwhile, buildings remain predominantly inefficient, accounting for 40% of final energy consumption and 36% of the total EU CO2 emissions. The ongoing energy transition brings the possibility of real-time energy resource management to building owners/managers and energy operators, with potential benefits for consumers, producers and the environment. To better tap into this potential, stakeholders must be able to continuously assess the energy performance of building energy systems and appliances, identifying areas where optimization services can be applied. Implementing this assessment and optimization capability requires real time monitoring and control of the building equipment and major energy consuming appliances. This functionality can be effectively performed by Internet of Things (IoT) enabled sensors and devices coupled to services that can assess and optimize the energy resources in buildings. The capability to analyze and optimize buildings energy resources and energy consuming equipment in useful time is not possible without employing Machine Learning (ML) techniques and big data infrastructures. This gives rise to ML Building Energy Management (BEM) Services that can be effective in an increasingly electrified and complex environment with energy flows between the grid, photovoltaic production, electric vehicles, storage batteries, building thermal capacity, and considering changing consumption patterns, occupants comfort, and highly variable user preferences.
Goals
The workshop follows the success of the 2021 edition (MLBEM 2021) co-located with ECML-PKDD’2021. The workshop gained strong interest, with an attendance between 15 and 25 participants, lively discussions after the talks, and an excellent invited talk. The workshop aims at filling a gap in the EU workshop panorama, providing researchers with a forum to exchange and discuss scientific contributions and open challenges, both theoretical and practical, related to the use of machine-learning approaches in building energy management. We want to foster joint work and knowledge exchange between the building's energy community, and researchers and practitioners from the machine learning area, and its crossing with big data, data science, and visualization. The workshop shall provide a forum for discussing novel trends and achievements in machine learning and their role in the development of scalable BEMs. It aims to highlight the latest research trends in machine learning, privacy of data, big data, deep learning, incremental and stream learning, and adversarial learning. In particular, it aims to promote the application of these emerging ML techniques to buildings energy management.
The workshop shall contribute to identify new application areas as well as open and future research problems related to the application of machine-learning in the building's energy field.
Find all about this workshop HERE